General comment about the edits - I realized soon after originally posting this article that the underlying numbers just didn't look right. Sure enough, I tracked down at least 2 mistakes in my math. I think I've got everything correct now, but I make no warranty. I reserve the right to continue to make mistakes, but I will do my best to avoid them.Before I get started, I just want to point out that the
player +/- stats page has been updated, and now includes all available games for the last 2 seasons.
-----------------------------------------------------------------------------------------------
In my
last few posts, I've be touting my new
HD Box Score MakerTM, which uses game play-by-play data to extract
a lot more info than a standard box score yields (all available HD boxes for the last 2 seasons
now posted).
In my never-ending quest to keep you, my only reader, ahead of the curve when it comes to basketball knowledge, I thought I'd start a series of posts using the data generated by my
HD Box Score MakerTM to learn a bit more about your Georgetown Hoyas.
To start, I thought I'd try to take on one of the questions that I raised at the end of my
intro post to HD box scores:
2. Was J. Rivers really that great of a defender? I'll look at the team's offensive and defensive efficiencies with each player on or off the court, to see if I can learn a bit more about the defensive side of things.
Analogous to
fielding defense in baseball, individual defense in basketball is not well-described by traditional basketball statistics. We can talk about team defensive stats (Def. Efficiency, DReb %, Def 2FG%, Def eFG%, Block %, etc.) with some confidence that we are able to describe what is actually taking place on the court, but the difficulty comes in attributing the individual defensive stops, rather than just taking a holistic view.
There are a few ways to tackle this problem:
- Watch each game, and chart each defensive possession for who was responsible for stopping (or allowing) the defense from a score. This has been advocated by Dean Oliver, the father (mid-wife?) of advanced basketball statistics - but, as far as I know, simply isn't available for college basketball games.
- Use the available box score data to estimate the number of stops each player makes, based on some rather large assumptions; one example of this metric is called Defensive Rating (also developed by Dean Oliver). This is similar to his Offensive Rating for individual players, which I, Ken Pomeroy and many others calculate, but uses less certain assumptions. Currently, I'm only aware of Henry Sugar at Cracked Sidewalks reporting Def. Ratings (example linked), although I'm sure there are others. I'll talk more about this stat below.
- Use the available play-by-play data to estimate the importance of each player to total team defense. Your first thought might be that we could use the play-by-play data to determine actual defensive stops by player, but we can't. The play-by-play doesn't tell us who is guarding whom, so we'd be back to the same assumptions that Dean Oliver uses. However, there is a simple analytical tool that we now have available to us: we now know how many points each team scored when any player was on (or off) the court. That is to say, we can calculate a team's offensive and defensive efficiency (points per 100 possessions) as a function of whether any player is on the court, and thereby look at what impact each player has on team offensive or defense.
At this point, I will explain some basic terms as a refresher, and also cover what's new here. All of this is explained in much more detail at the web pages linked to the right under "Tempo-Free Stats 101." Feel free to skip ahead if this is all familiar.
Possession-based (tempo-free) statistics is a concept in basketball going back
at least as far as Frank McGuire, and is useful for comparing players and teams who operate at different paces, or speeds of play. A possession ends either by a made basket (including
some made FTs), a turnover or a defensive rebound - that's it, that's the list. Offensive rebounds don't create new possessions, only prolong the current one. If you use this definition, two teams will end up with either the same number (± 1) of possessions at the end of any game; since possessions go back-and-forth, it must be so. The equation for estimating total possessions per team per game is floating in various forms around the internet, but I will add to the clutter:
Possessions = FGA + 0.44 * FTA - OReb + TO
Since this formula is only an estimate of the actual number of possessions, I find that it is best to solve for each team, then take the average. Any team's (or player's) stats should be instantly comparable to any other with a per-possession system, since what is expressed is team (or player) efficiency rather than counting stats.
Offensive (and
defensive)
efficiency is a team statistic expressed in the units of points per 100 possessions (why per 100 possessions? so there aren't so many digits to the right of the decimal). This statistic is rather simple to calculate, once you've worked out how many possessions have been played with the equation above.
Ken Pomeroy, one the populists of tempo-free stats, has an additional version of this stat, called
adjusted off. (or def.) efficiency. Here, he attempts to weight points per possession based upon quality of opposition.
Offensive rating (as mentioned above) was created by Dean Oliver in an attempt to better rate individual basketball players on offense. The calculation of this stat is not simple - I've had people ask me in the past for the equation, but it's actually a bunch of equations (see
this book for details). In simple terms, it is the ratio of
points produced (
not scored) by any player, divided by
possessions used (
not played), with both of these terms estimated from normal box score data. It is a tempo-free statistic, since it is expressed in points per (100) possession. Since players should be credited for assists and offensive rebounds as well as actual points scored, this rating is just an estimate of actual player worth, but the underlying assumptions are well thought out (you'll have to trust me, or read the book).
Defensive rating is an attempt to estimate the contribution of each player to the team's defensive efficiency. It is calculated as team defensive efficiency, plus one-fifth of the difference between team defensive efficiency and individual player stops per 100 possessions played. Player individual stops are estimated from the number of blocks, steals and defensive rebounds each player has, plus some team stats. Since it is not a simple ratio, it is more like being graded on a curve, such as that it is limited to the range of 80% - 120% of team defensive efficiency. So, a player who literally refused to play defense (e.g. Donte Greene) could score no worse than 80% of his team's efficiency. I would describe this stat as a very rough estimate of actual defensive worth . . .
. . . which leads me back to the point of this post (there really is a point). Now that I have access to the play-by-play of most G'town games, can I use this to better estimate the defensive contribution of each player, on a possession-basis? If so, we could finally talk about the overall value of a player to his team, rather than just his offensive contribution. The play-by-play shows who was on the court at any point during the game, so we can assign partial credit to each player for how well the team plays at both ends while he's in the game; likewise, we can see if the team plays better or worse when he leaves. This is really just applying the concept of
plus-minus and Net/40 (or
Roland rate), but rather using possession info to speak in tempo-free terms, rather than per-minute.
To explain explicitly here, I've taken each player, and added up the points that G'town scored and allowed while he was on the court. and how many were scored and allowed when he was off the court. In each case, I also know how many offensive or defensive possessions he participated in, so I can divide each point total by respective possessions (times 100) to find the team's offensive of defensive efficiency while he was on or off the court. Then, I find the difference between on- and off-court efficiency (either off. or def.) and add that to the team's efficiency.
For example, to calculate Jessie Sapp's Net Offensive Efficiency:
Jessie Sapp played
1171 offensive possessions, and the Hoyas scored
1298 points while he was on the court.
[1298 / 1171 x 100 = 110.8 Off. Eff. on-court]
He sat for
561 offensive possessions, and the Hoyas scored
585 points while he sat.
[585 / 561 x 100 = 104.3 Off. Eff. off-court]
So Jessie Sapp's
Net Off. Eff. is equal to:
(Off. Eff. on-court - Off. Eff. off-court) + Team Off. Eff. =
(110.8 - 104.3) + 108.7 = +6.5 + 108.7 = 115.2 pts/100 possessions
Before I jump into the defensive analysis, I first want to see if my idea that
player-based on-court / off-court net efficiency correlates to individual player rating holds water. To do this, I'll take a look at each player's net offensive efficiency versus individual offensive rating for last season.
One last point before I go on, the individual player ratings here won't match exactly with what either Pomeroy or I post for season totals. Since I don't have play-by-play data for all games, I re-ran the player ratings using box score data only for games that also had p-b-p data [to see which games are missing, go to the
player +/- page].
Let's take a look (
as always, click any image to enlarge):
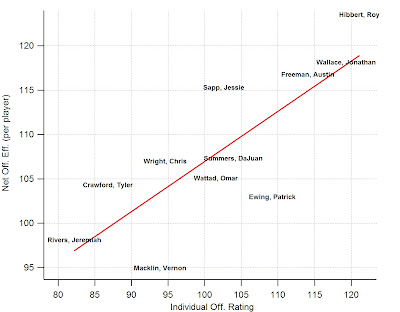
This seems to work quite well! Players in the upper right of the graph are the best offensive players by either metric, while players in the lower left are not carrying their weight. The red line is a linear fit to all the data (r = 0.81), excluding Bryon Janson, who just doesn't have enough playing time to generate meaningful stats. The slope of the line is about 0.55, significantly less than 1, which is actually to be expected. The Net Team Efficiency stat doesn't completely isolate a player from his teammates in the way that Off. Rating attempts to do; since there is variability player-to-player, the range of the Net. Team Eff. stat gets compressed - a bad offensive player surrounded by good players will look better than he is.
The strong correlation indicates that the two statistics are highly coupled (co-variant). Individual offensive rating is a fairly
well-accepted statistic, and it seems to do a good job of measuring how important a player is to team efficiency. Of course, the converse should also be true - team offensive efficiency as a function of each player on or off the court is a good measure of individual offensive value.
Moreover, and here's where I may be stretching the statistics a bit, the scatter plot can tell us a bit more: if a player is above the line, he makes the team more efficient than expected based upon Off. Rating (i.e. the player is underrated by Oliver/Pomeroy/etc.) while if he is below the line, he is overrated. Keep in mind that there are considerable uncertainties for the data on both axes that are not shown or even calculated, because that would make my life a lot harder. But it looks like Jessie Sapp and Roy Hibbert were underrated offensively last season, while Patrick Ewing Jr. and Vernon Macklin were overrated.
Now, let's now take a look at the defensive side. The math as the same as presented above, just looking at defensive possessions now.
Things here are not so clear-cut as for offense. There is poor correlation between the two data sets (r = 0.11), so I've just thrown a 1:1 line onto the chart. Note that both axes have their scaling reversed (they get smaller as you head away from the origin), since a lower defensive rating or efficiency is better. Again, players in the upper right corner are the best defenders, those in the lower left are the worst.
One thing that I notice immediately is that the scaling for the two statistics is much closer to 1:1 than for offense. We already expect that Net Def. Rating should be compressed since we can't isolate individual players, only their effect on the team while on the court. But here, the Def. Rating stat shows about the same scaling meaning either a) there isn't as large a difference between a good and bad defensive player as there is for an offensive player, or b) the Def. Rating stat isn't able to isolate individual defensive skills.
To take this a bit further, we demonstrated above that the Net. Efficiency methodology for offense seems to work quite well in correlating to a "good" measure of offensive prowess, albeit on a somewhat compressed scale. Since the method is identical for defense and offense, there's no reason to expect Net Efficiency to stop working for defense. Therefore, it could be argued (I just did) that Net. Def. Eff. is a better measure than Def. Rating.
If you've not read Basketball on Paper, you should probably just skip over the next paragraph.
Digression: Before I go on, I should point out that I'm using a slightly modified version of D. Oliver's Defensive Rating calculation. His formula estimates defensive stops in two parts, and the second has a necessary assumption that he, himself, acknowledges to be poor with regards to position-specific uncredited stops. Since centers get a disproportionate number of stops by way of blocks, they tend to be overweighted by his formula (for reasons far too obscure to explain here). I've added a simple weighting factor, based on Steals/(Steals+Blocks) to correct this. For this data set, the effect of this correction ranges from -2.7% for Roy Hibbert to +2.3% for Jonathan Wallace and Chris Wright. (n.b. - The weighting factor is based on Oliver's own data). Returning to the scatter plot, once again we can find over- and underrated players by using the fitted line.
Now, Tyler Crawford and Chris Wright are the most overrated defenders, while Jonathan Wallace (!), Roy Hibbert and Jessie Sapp are underrated. Of course, that only looks at the comparison between two poorly correlated stats; the real take-home message is that Hibbert, Sapp, DaJuan Summers and Patrick Ewing anchored last year's excellent defense, just as Hibbert, Wallace and Austin Freeman were the most effective on offense.
And to answer the question that started this whole thing - yes, Rivers was a good defender, but not extraordinarily so, and not as important as Hibbert, Ewing or Summers. In fact his stats are not obviously better than either Sapp's or Wallace's(!). I will speculate that Rivers was the best on-ball defender as a guard, but Wallace and Sapp were more sound within the defensive schemes used last year (
I just made that up). And I'm not sure why Omar Wattad looks like
Gene Smith on this plot, but I'll guess it's just the result of a small sample size (n = 58 def. possessions).
Finally, we can combine the offensive and defensive metrics on a single plot, to get a rank of the overall value of each player. Here, I'm simply taking the average of Off. Rating & Net. Off. Eff. for the y-axis, and the average of Def. Rating & Net. Def. Eff. for the x-axis. Here, I'm hoping that the averages of two measures of the same variable come closer to describing its true character than either measure on its own.
This plot is a bit more complicated, as I'm trying to convey a lot of information.
Again, you'll need to re-jigger yourself to the axes. Offense is on the left-axis, with up equaling better performance; defense is on the bottom axis, but still with reversed scaling, so that right equals better performance. Players in the upper right are most valuable, players in the lower left are least valuable; those closer to the upper left have more value on offense, those down and to the right have more value on defense. This last bit looks to be well correlated with expectation, as the two players best know for offense rather than defense (Wallace & Freeman) show up right about where you'd think.
The size of the names are now scaled by possessions played, so Omar Wattad's newly-discovered value as an all-world defender is now tempered by the fact that he rarely plays. DaJuan Summers somewhat swallows up Patrick Ewing because of this, but I think you can still make them out.
Finally, the series of diagonal lines (
isopleths) on the chart mark show contours of total player value (i.e. the difference between off. and defensive worth). For example, Jessie Sapp's off. worth = 108.9 and his def. worth = 89.8, so you'd expect that he'd provide +19.1 (= 108.9 - 89.8) pts./100 poss. to the team. Meanwhile, Austin Freeman's off. worth = 115.4 and his def. worth = 95.8, so you'd expect that he'd provide +19.6 pts./100 poss. That is, Sapp and Freeman were essential equally valuable to last year's team on a per possession basis, although they did it in different ways. Because they are rated at equal value, they both lie on about the same position relative to the diagonal lines.
Moreover, if you had a team of players all equally efficient overall as Sapp and Freeman, you'd expect the overall difference in team Off. Eff. and Def. Eff. to be around +19 pts/100 poss. FWIW, last year,
G'town's efficiency difference was +18.8 (raw, not adjusted), good enough for a #2 seed in the NCAA tournament.
Here's a summary table of off., def. and total player worth for all the players:
Off. Def. Total
Hibbert, Roy 122.3 90.2 32.1
Wallace, Jonathan 118.7 94.0 24.7
Freeman, Austin 115.4 95.8 19.6
Sapp, Jessie 108.9 89.8 19.1
Ewing, Patrick 106.1 90.5 15.6
Summers, DaJuan 105.6 90.6 15.0
Wattad, Omar 103.3 88.3 14.9
Wright, Chris 100.8 93.2 7.6
Crawford, Tyler 95.6 95.4 0.1
Rivers, Jeremiah 90.1 94.4 -4.3
Macklin, Vernon 94.4 100.0 -5.6
We will miss Roy Hibbert. On a team that was
ranked 7th by Ken Pomeroy after the NCAA tournament, Mr. Hibbert was the best Hoya player on the court by a large margin.
Next comes the gang of five (+1), who were the other important contributors to the Hoya's success; in
very rough order of importance: Wallace, then Freeman and Sapp, then Summers and Ewing. Wattad sneaks in right behind this group, despite his few possessions (who knows, maybe the whole point of this article was to find a new player to champion; after all,
the last time went so well).
By my reckoning, the two soon-to-be-transfers were not helping much last season. In the end, Rivers' defense just couldn't make up for his offensive woes, while Macklin struggled at both ends of the court. I never gave much thought to Macklin's defense, but he was easily the worst defender on the team by my numbers. Does that seem right? Tyler Crawford struggled with his outside shooting last year (3-22 3FGs), and wasn't able to make up for it with great defense.
There's one returning player that I haven't mentioned - Chris Wright. He missed the majority of the season with a foot injury, and ended up with a little more than half of Macklin's total time played. After struggling early in the season, he seemed to have a breakthrough in the 2nd half against Derrick Rose and Memphis, only to go down 2 games later. And while he played in 5 post-season games after getting healthy (3 in the BET, 2 in the NCAA), I only have the Pitt game in the BET finals in this analysis, since I don't have p-b-p for the other 4 games. From looking at the box scores, 3 of the 4 games I'm missing (Villanova, UMBC, Davidson) were among his best.
What I'm saying is that I think Wright is being undervalued here, not because there's anything wrong with the analysis, but because his underlying data doesn't do him justice. I hope I'm right.